Revolutionizing Rural Address Geolocation with GeoResonanceGPT
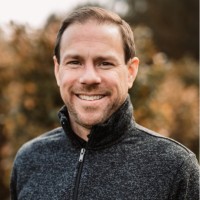
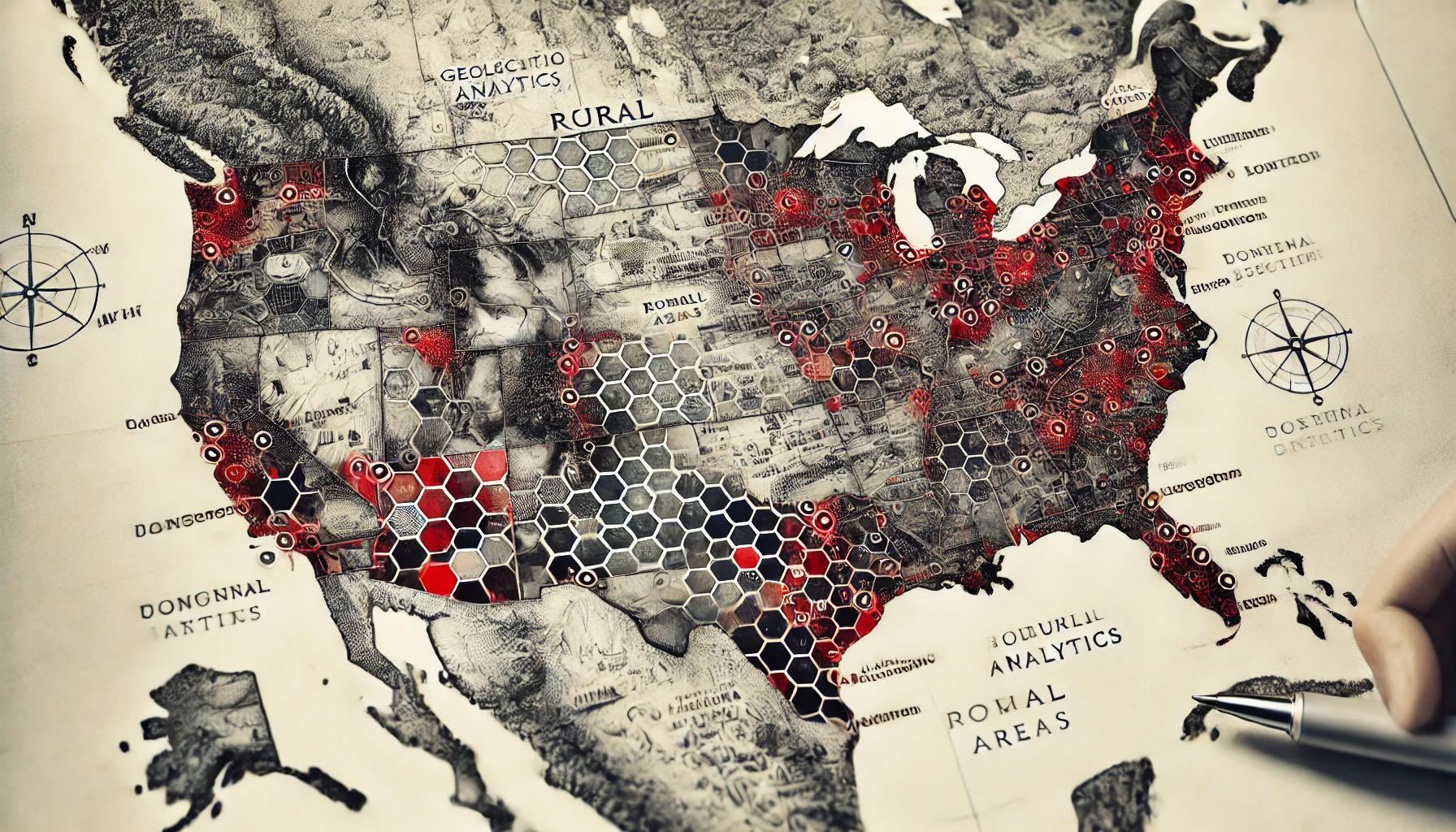
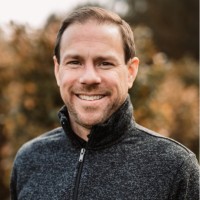
CloudRaven’s GeoResonanceGPT initiative offers a transformative framework for enhancing the U.S. Census Bureau’s Master Address File (MAF) coverage in rural and remote areas. By integrating public-domain datasets, Census-provided data, machine learning (ML), computer vision, multi-modal Large Language Models (LLMs), and Retrieval Augmented Generation (RAG) from a knowledge graph, we deliver a high-precision, scalable solution that focuses on the most difficult geolocation challenges: non-city-style addresses in rural areas, such as American Indian areas, Puerto Rico’s mountainous interior, remote Alaska, and other “data deserts.”
Despite the Census Bureau’s advanced automated change detection and building footprint extraction capabilities, rural and remote address geolocation remains a significant challenge. Gaps persist where non-city-style addresses, cultural nuances on American Indian reservations, incomplete parcel coverage (in Alaska or Puerto Rico’s interior), and hidden or informal housing units evade conventional data sources. Addressing these last-mile scenarios requires innovative reasoning and validation techniques beyond what currently exists.
GeoResonanceGPT Concept
GeoResonanceGPT supplements current ML-driven methods with a multi-modal LLM that reasons over a knowledge graph for geolocation use cases when generating annotations and scoring inputs. This integration format enables:
-
Entity Extraction & Relationship Modeling: Convert imagery, vector data (e.g., TIGER/Line), textual descriptions, and partner datasets into a structured RDF graph. Encode cultural norms, land use, and administrative rules to form a holistic semantic network.
-
RAG with Knowledge Graph: The LLM queries the graph for domain-specific facts, reasoning through subtle scenarios—e.g., confirming if a structure is a ceremonial Hogan or identifying a secondary basement apartment invisible at first glance.
-
Human-in-the-Loop Validation: Only the toughest cases reach experts, who leverage semantic queries and explainable inference paths. Their feedback updates models, refining performance iteratively.
CloudRaven's GeoResonanceGPT initiative represents a significant step forward in addressing the complexities of rural and remote address geolocation, ensuring that even the most challenging areas are accurately represented in the U.S. Census Bureau's Master Address File.