Beyond The Black Box: Explaining and Auditing AI Models for Better Insights
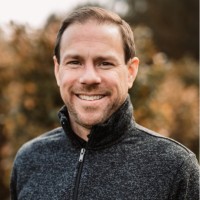

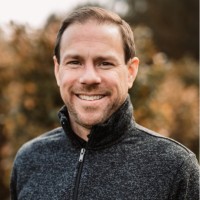
Much of AI’s transformative power lies in its ability to uncover hidden patterns within vast datasets. Yet, as algorithms grow more complex—especially Generative AI models that produce text or images—it becomes increasingly difficult for stakeholders to understand how decisions are made. CloudRaven Labs addresses this challenge head-on by emphasizing model explainability and auditing as cornerstones of our AI offerings.
Why Explainability Matters
An opaque model can erode user trust, even if its predictions are highly accurate. Explainability goes beyond revealing raw inputs and outputs; it clarifies why certain features or data signals influenced an AI decision. For stakeholders—ranging from legal teams to frontline analysts—transparency translates into:
-
Risk Mitigation
Regulatory bodies often demand proof of non-discrimination. An auditable model can demonstrate fairness and compliance with anti-bias guidelines. -
Informed Decision-Making
When domain experts know which factors contribute most to an AI-driven insight, they can blend their own expertise into final decisions with greater confidence. -
Increased Adoption
Teams across an organization—particularly those less familiar with AI—are more likely to embrace new tools if they can see clear logic behind the outputs.
CloudRaven’s Approach to Explainable AI
1. Model Anatomy Breakdown
- Layered Architecture: Each component within our AI pipeline (data ingestion, preprocessing, feature engineering, ML model, and final output generation) is modular and documented.
- Traceable Feature Importance: For tasks like job listing matching or grant ranking, we provide a feature importance score that highlights which data attributes (keywords, semantic embeddings, etc.) drive the final recommendation.
2. Auditable Workflows & State Machines
- Comprehensive Logs: Our state-machine approach generates a digital paper trail of each data point as it moves through the pipeline.
- Actionable Audits: Whether you need to investigate a suspicious data point or validate how a grant scored the highest, our system’s event logs offer granular visibility.
3. Human-in-the-Loop Validation
- Expert Review: CloudRaven’s platform prompts domain experts to review or override AI outputs at critical junctures, maintaining accountability and quality control.
- Feedback Integration: Any edits or annotations from humans feed back into the pipeline, refining future AI outputs in an iterative, continuous improvement cycle.
Real-World Use Cases
-
Grant Eligibility Reviews
- Explainable Ranking: Government agencies or nonprofits can see why certain grants rank higher (e.g., emphasis on aging populations vs. climate initiatives), ensuring alignment with policy objectives.
- Transparency for Stakeholders: When presenting a proposal to local councils, teams can justify the AI’s top-choice grants with clear, data-backed reasoning.
-
Job Matching & Resume Screeners
- Bias Audits: HR leaders can monitor whether certain skills or demographics are unintentionally deprioritized.
- Confidence Scores: Job seekers benefit from understanding how certain aspects of their resume (e.g., relevant experience, skill keywords) matched with a given listing.
-
Community Wellbeing Assessments
- Interpretable Indexing: Municipalities can determine which factors (crime rate, education level, healthcare access) had the greatest weight in a wellbeing score, informing targeted interventions.
- Public Accountability: Explaining why certain neighborhoods rank higher or lower fosters public trust and encourages data-driven discussions.
Benefits for CloudRaven Labs and Clients
-
Faster Issue Resolution
When anomalies arise, an auditable pipeline helps pinpoint root causes—be it a data anomaly, a misunderstood algorithmic step, or a misapplied weighting. -
Cross-Functional Collaboration
Clear data lineage and interpretability foster cooperation between technical teams, domain experts, and organizational leadership. -
Competitive Differentiation
As AI regulations tighten, many organizations seek explainable solutions. CloudRaven’s approach meets this demand, positioning us as a trusted partner. -
Future-Proofing
Explainability isn’t just a buzzword—it’s becoming a standard expectation. By embedding it into our platform, we future-proof projects against emerging guidelines and best practices.
Looking Ahead
Over time, Generative AI models will grow more sophisticated, tackling bigger challenges with broader datasets. For CloudRaven Labs, ensuring these models remain transparent and auditable is not just an option—it’s a necessity. Our ongoing commitment to explainability allows our clients to harness cutting-edge AI technologies without compromising on ethics, compliance, or user trust.
© 2024 CloudRaven Labs. All rights reserved.